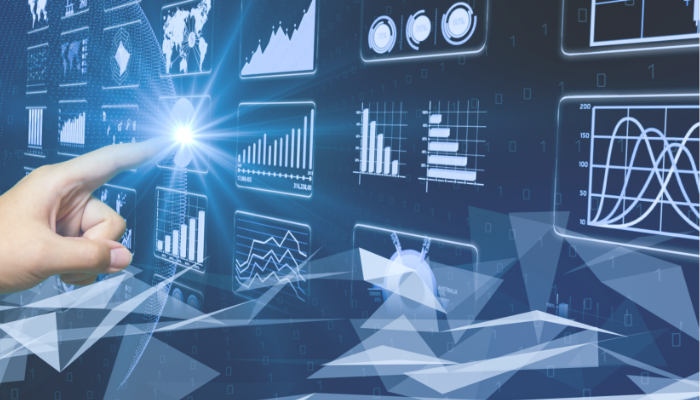
The last quarter Financial Results of many Lending institutions in India have raised very serious concerns about the process , methodology and management of the hyper growth lending industry in India.
Yes, the Indian lending industry has experienced remarkable transformation in the recent years, driven by technology, regulatory measures, and rising demand for efficient credit solutions. Despite these advancements, challenges like efficient debt collection practices, credit risk assessment, and financial inclusion remain significant for small and medium enterprises (SMEs) and micro borrowers.
The Last Quarter Financial results of many Lending institutions in India have raised very serious concerns about the process, methodology and management of the hyper growth lending industry in India.
By leveraging the power of Analytics and Ai and with integration of consortium data, account aggregators, regulatory frameworks, alternate data, dynamic debt collection insights, the Indian lending ecosystem can achieve a robust growth with adequate risk mitigation measures in place
The Role of Consortium Data in Debt Management
Traditional Challenges in Debt Management
Indian lenders often depend on traditional credit data such as basic credit scores, repayment history, and financial statements which can be insufficient for managing the lending process in a fast changing lending ecosystem like India, especially for small and medium enterprises (SMEs) and individuals with limited credit histories and Micro Financing
Consortium Data: A Game Changer
Consortium data refers to information shared among multiple lenders and financial institutions. By pooling transactional data, customer repayment behavior, customer responses, trade credit records, and payment histories, it provides a comprehensive 360-degree view of a borrower’s financial health.
Impact on the Lending Industry:
- Enhanced Risk Insights: Consortium data enables lenders to assess a borrower’s Repayment behavior more effectively by analyzing a comprehensive range of financial behaviors.
- Reduced Information Asymmetry: Access to shared data helps lenders identify potential risks and opportunities with greater precision, leading to improved Debt Management /Collection decisions.
- Tailored Lending Solutions: Comprehensive data empowers lenders to design customized credit products for SMEs, microenterprises, and underserved populations.
Use Case: SME Lending
In the SME lending space, consortium data proves particularly transformative. Indian SMEs often operate in sectors with fluctuating cash flows. Consortium data enables lenders to distinguish between temporary liquidity challenges and chronic financial instability, allowing them to offer tailored Debt Repayment solutions accordingly.
Account Aggregators: Bridging the Data Gap
The Account Aggregator (AA) framework, introduced by the RBI, facilitates secure, consent-based financial data sharing, creating a centralized borrower information repository.
Advantages of the AA Framework:
- Streamlined Data Access: Account Aggregators (AAs) consolidate data from banks, insurance companies, and mutual funds, providing lenders with a unified view of a borrower’s financial profile.
- Improved Financial Inclusion: By facilitating secure and convenient data sharing, AAs enable underserved populations to access formal credit channels.
- Reduced Operational Overheads: Centralized data access minimizes redundant documentation, enhancing efficiency for lenders.
Use Case: Retail Lending
Retail borrowers often face challenges repayment cycles because of external market conditions. The AA framework data appended by individual repayment behaviour data can accurately forecast the next payment action of the borrower and provides lenders with a comprehensive action plan to recover the debts.
Leveraging Real Time Debt Management/Collection Data
With the democratization of the Lending Industry, Lenders no longer have the advantage of mitigating the Risks through a Robust Credit Scoring process. In such a scenario, the only solution for lenders is to build a efficient debt collection process by leveraging Technology and Data
The Case for Real Time Debt Collection Data /Analytics
Since Debt Collection is a 30 day cycle business, it is extremely critical that the Lenders undertake dynamic and customized debt recovery process in order to achieve a cost effective, automated and analytics driven debt collection process
- Real Time Digital Communication data : By leveraging the multi lingual conversational engines/bots, lenders can collect very insightful data of the payment behavioral change of the borrowers
- Borrower’s sentiment data : By collating and analyzing the borrower’s conversational data ( Call center conversations, Disposition Codes, Legal notices reply etc) , lenders can get real hidden insights on “Willingness” to pay behavioral dynamics of the borrowers
- Legal Management data : The near real time data from the legal institutions can give real insights on the potential risks with respect to a borrower
- Geolocation Data: This provides valuable insights into the fraudulent activities, collection operational viability and market dynamics for borrowers, particularly in underserved or remote regions.
Importance of Collection Data
Debt collection data offers valuable insights into borrower behavior post-loan disbursement. Metrics such as repayment patterns, delinquencies, and collection efforts play a crucial role in informing future credit decisions.
Integration with Gen AI:
When integrated with Generative AI (Gen AI), debt collection data can:
- Predict Defaults: Detect early warning signs of delinquency for proactive intervention.
- Optimize Collection Strategies: Customize collection approaches based on borrower-specific behaviors.
- Refine Credit Models: Enhance risk models by incorporating real-world outcomes from collection data.
4. Leveraging Alternate Data
The Case for Alternate Data
In a country like India, where traditional credit data remains limited for a substantial portion of the population, alternate data offers invaluable insights. Key examples include:
- Utility Bill Payments: These serve as reliable indicators of a borrower’s financial discipline and ability to manage recurring expenses.
- Telecom Data : The telecom data shall provides insights on recent activities of the borrowers
- Social Media Metrics: These metrics reflect business activity and consentient, offering insights into Repayment behavior and operational health.
5. Integration Across the Ecosystem
Unified Lending Platforms
Combining consortium data, account aggregators, regulations, alternate data, real debt collection data, and Gen AI requires unified platforms to:
- Streamline Data Access: Offering a single interface for lenders.
- Ensure Compliance: Adhering to RBI regulations and data privacy norms.
- Enhance Scalability: Managing high transaction volumes efficiently.
The Role of APIs:
Application Programming Interfaces (APIs) facilitate seamless data exchange between lenders, regulators, and other stakeholders. This ensures real-time updates and a cohesive ecosystem.
The Role of Regulators and the RBI in Shaping Debt Management
6. Integration with RBI Data Systems:
Integrating lending platforms with RBI’s data systems provides lenders with access to critical macroeconomic indicators, borrower default histories, and compliance records. This comprehensive integration enhances transparency, minimizes systemic risks, and facilitates informed decision-making across the lending landscape.
7. Gen AI in Real-Time Credit Monitoring
Generative AI models can analyze vast volumes of alternate data to detect patterns and predict Repayment behaviour effectively. For example, an SME’s foot traffic data or online engagement metrics can act as valuable proxies for gauging sales performance and revenue trends.
Generative AI (Gen AI) has the potential to transform every stage of the lending lifecycle. From application processing to credit assessment and debt recovery, AI-powered models bring unparalleled accuracy, efficiency, and scalability to the industry.
With real-time monitoring capabilities, Gen AI can detect subtle changes in borrower behavior, such as declining transaction volumes or irregular payment patterns. This allows lenders to act proactively, mitigating risks before they escalate.
Conclusion
The Indian lending industry stands at the cusp of a technological revolution. Integrating consortium data, account aggregators, alternate data, and Generative AI can redefine credit risk assessment, debt recovery, and financial inclusivity. These advancements not only foster a robust ecosystem but also ensure scalability, efficiency, and inclusivity in the evolving financial landscape.